Model registry governance is the practice of managing, tracking, and controlling machine learning (ML) models across their lifecycle. A model registry is a centralized system where organizations store versions of models, along with metadata, performance metrics, and deployment status. Model registry governance ensures these records are organized, verified, and aligned with internal and external compliance requirements.
Model registry governance matters because organizations are increasingly under pressure to prove the quality, fairness, and safety of their AI systems. Good governance helps risk and compliance teams track who created a model, what data it was trained on, how it performs, and whether it meets regulatory standards. Without it, organizations risk losing control over critical AI assets, facing security vulnerabilities, and failing audits.
Why model registry governance is critical
According to a recent report by IBM, 85% of companies adopting AI say they struggle with managing model risks. Yet only 20% feel confident in their model management practices. This gap creates major vulnerabilities, especially as AI regulations like the EU AI Act and standards like ISO/IEC 42001 are coming into force.
“Seventy-eight percent of companies report that AI model failures have directly impacted their business operations over the past year” (Gartner survey, 2024).
A model registry provides a structured place to track models, but without governance, the registry becomes just another database. Model governance creates the policies, review workflows, and accountability needed to make the registry useful and reliable.
Key components of model registry governance
Model registry governance combines technical controls with organizational policies. Some of the essential components include:
-
Version control: Every model version must be tracked, along with the data and code that produced it.
-
Metadata management: Registries should require and validate important metadata such as model type, input features, target variables, training datasets, performance metrics, and explainability reports.
-
Approval workflows: Models should go through human reviews before they are moved to production environments. The workflows should include risk assessments.
-
Access controls: Only authorized individuals should be able to register, update, promote, or deprecate models.
-
Audit trails: Registries must keep complete logs of model changes, deployments, rollbacks, and retirements.
-
Monitoring integration: Production models must be linked to monitoring systems that report performance drift, bias, and reliability issues.
These elements help organizations create transparency and build trust internally and externally.
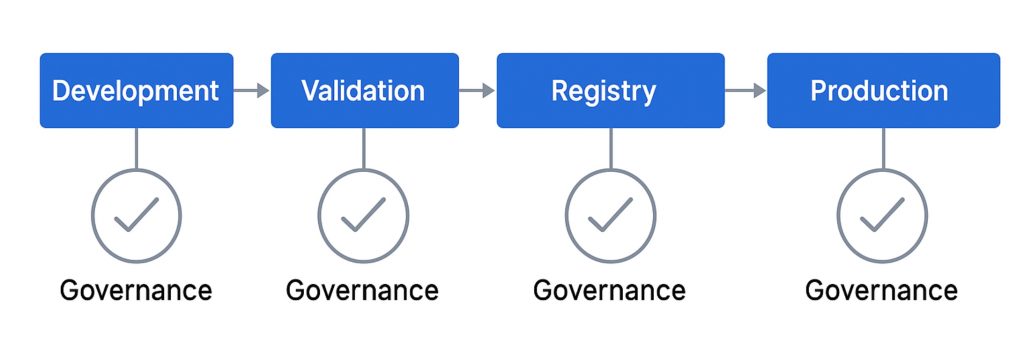
Best practices for model registry governance
Strong model registry governance depends on following clear best practices. Organizations that are serious about AI compliance usually start with small steps and then expand over time.
-
Standardize model documentation: Require teams to fill out structured documentation templates before a model is accepted into the registry.
-
Use model cards and datasheets: Encourage the use of model cards and datasheets for datasets to maintain consistent metadata and transparency.
-
Define promotion criteria: Set objective thresholds for performance, fairness, and robustness that a model must meet before moving from staging to production.
-
Automate governance checks: Build automated validations into the registration pipeline to catch missing fields, metadata errors, or expired approvals.
-
Map models to risks: Maintain a risk register that links each model to known risks, regulatory requirements, and mitigations.
Taking these steps improves operational efficiency and reduces the chances of costly mistakes during audits or incidents.
Frequently asked questions
What is a model registry?
A model registry is a centralized database where machine learning models are stored, versioned, and managed across their lifecycle.
Why do companies need model registry governance?
Companies need governance to ensure that models are safe, compliant, traceable, and ready for audits. Good governance also reduces operational risks and improves collaboration between teams.
How does model registry governance relate to AI regulations?
New regulations like the EU AI Act and standards like ISO/IEC 42001 require organizations to maintain accurate records of their AI models, assess risks, and monitor model behavior. A strong registry governance process supports these requirements.
Can model registry governance be automated?
Parts of it can be automated, such as metadata validation, risk scoring, and approval workflows. However, human reviews are still necessary for ethical, legal, and safety evaluations.
What tools are commonly used for model registries?
Popular tools include MLflow Model Registry, Weights & Biases Model Registry, Amazon SageMaker Model Registry, and Azure Machine Learning Model Registry.
Summary
Model registry governance is a foundational pillar for responsible and compliant AI development. It provides the structure, processes, and accountability needed to manage machine learning models safely and transparently. Organizations that invest in model registry governance early are better prepared to face regulatory demands, avoid operational risks, and build trust with their customers and regulators.