Model assessment reports are documents that evaluate machine learning models against predefined criteria such as performance, fairness, explainability, robustness, and regulatory compliance. These reports are typically generated before a model is released into production and are updated periodically as part of continuous monitoring. A strong model assessment report creates transparency and serves as a foundational record for audits, risk management, and external reviews.
Model assessment reports matter because they provide formal evidence that AI models are evaluated and approved based on objective criteria. Risk and compliance teams need these reports to demonstrate responsible AI practices to auditors, regulators, and customers. Without documented assessments, organizations face serious exposure to operational, legal, and reputational risks.
Why model assessment reports are critical
A survey by McKinsey found that only 21% of companies applying AI conduct consistent risk assessments before launching models. This creates blind spots that could lead to model failures, biases, or compliance violations.
“Sixty-two percent of organizations report at least one AI incident or failure that required a formal response in the past two years” (World Economic Forum, 2024).
Model assessment reports address these issues by ensuring each model is scrutinized properly before it affects users, customers, or critical business processes. As standards like ISO/IEC 42001 set clear expectations for AI governance, structured reports become even more important.
Key elements of a model assessment report
Model assessment reports are built around several important elements. These elements make the report usable not only for technical teams but also for risk, legal, and executive audiences.
-
Model overview: A summary that includes the purpose of the model, target variables, and intended users.
-
Performance evaluation: Metrics such as accuracy, precision, recall, F1 score, ROC-AUC, or domain-specific benchmarks.
-
Fairness analysis: Checks for demographic bias, disparate impact, and subgroup performance differences.
-
Explainability evaluation: Description of explainability methods used, such as SHAP values or LIME explanations, and whether explanations meet regulatory and business expectations.
-
Risk analysis: A breakdown of identified risks such as data bias, adversarial vulnerabilities, or ethical concerns.
-
Regulatory compliance: Documentation that the model aligns with applicable laws and standards, such as GDPR, HIPAA, or the EU AI Act.
-
Validation and testing: Description of test sets, validation methods, and external validations if applicable.
-
Sign-off section: A record of stakeholders who reviewed and approved the model assessment.
A well-organized report helps simplify audits, supports better decision-making, and strengthens internal accountability.
Best practices for creating model assessment reports
Building good model assessment reports requires consistency and discipline. Following best practices helps organizations maintain quality and avoid unnecessary confusion later.
-
Standardize templates: Create a company-wide template for all model assessments to ensure consistent quality and completeness.
-
Include human review: Automated validation is useful, but human oversight is essential for ethical and contextual judgments.
-
Tie assessments to risk levels: Require more detailed assessments for higher-risk models, such as those affecting healthcare, finance, or personal data.
-
Track versions: Update the report every time the model is retrained, modified, or redeployed.
-
Store reports securely: Keep reports in an auditable repository that risk and compliance teams can easily access during evaluations.
Starting with these steps reduces future disputes and supports better model governance.
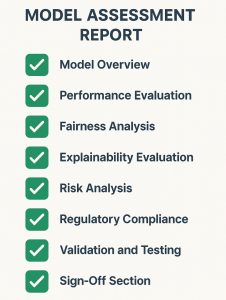
Frequently asked questions
What is a model assessment report?
A model assessment report is a formal document that evaluates a machine learning model’s performance, fairness, risks, and compliance with internal and external standards.
Why are model assessment reports important for AI governance?
They create structured evidence that models have been properly tested, reviewed, and approved before use. This documentation supports compliance with regulations and industry standards and protects organizations from operational, legal, and reputational risks.
Who should review a model assessment report?
The review should involve technical leads, compliance officers, risk managers, and sometimes external auditors depending on the model’s criticality.
How often should model assessment reports be updated?
Reports should be updated whenever a model is retrained, materially changed, or when new regulatory requirements emerge that affect the model’s operation.
Are there tools that support model assessment reporting?
Several platforms such as MLflow, Weights & Biases, and Azure Machine Learning offer capabilities to automate parts of the assessment process, but human oversight remains necessary.
Summary
Model assessment reports are essential tools for building trustworthy and compliant AI systems. They capture the vital information needed for transparent governance, regulatory readiness, and operational reliability. Organizations that treat model assessment reports seriously create a stronger foundation for sustainable AI success.